Computational Theories of Mindfulness
By Liza Tatishev
Mindfulness involves non-judgmental moment-to-moment self-awareness of physical and mental states– it is the ability to be fully conscious and aware. Within the past decade, scientific interest in mindfulness practices has increased. Mindfulness has a long history: originating over 2,500 years ago in Indian Buddhist tradition [5]. By the mid-twentieth century, mindfulness became secularized and incorporated into psychological intervention methods to improve emotional well-being [6]. Today, mindfulness-based intervention methods are recognized as successful approaches in treating a variety of psychological disorders, including depression, anxiety, and addiction, thus making it clinically significant.
A more precise understanding of mindfulness can benefit the refinement of mindfulness-based intervention methods. The field of computational neuroscience offers a method to examine the neuroscience of mindfulness. In order to approach the neuroscience of mindfulness computationally, it is important to first clarify the physical reality of the brain that is being represented via algorithms.
Contents
Psychological Mechanisms
As a psychological intervention method, mindfulness practices– such as Tibetan Buddhist imagery meditation [7], Zen meditation [8], and Kundalini yoga [9]– are grounded in training and refining attention skills. Attentional control is a key cognitive process of executive functioning (also referred to as cognitive control) necessary for emotional and behavioral regulation [10].
Cognitive control
Mindfulness training has been shown to enhance cognitive control in children and adults [11]. On a phenomenological level, the process of mindfulness training engages with the self-regulation of cognitive states that are interrelated with attentional networks.
Within cognitive psychology, attention consists of sub-processes (including alerting, sustained attention, and conflict monitoring) that underlie the ability to attend to stimuli. Higher self-reported mindfulness is related to higher measures of more stable sustained attention [12]. Furthermore, longitudinal studies have found that engaging in mindfulness practices correlated with improvement in sustained attention tasks over the course of intervention [13].
Sustained attention recruits attentional control functions, while inhibiting proponent, but non-relevant responses [14]. Research has demonstrated that mindfulness may improve performance on attentional control tasks, however experimental designs should be improved to remove discrepancies in the field [15].
Evidence for mindfulness practice improving sustained attention and attentional control processes exists. Enhanced cognitive control network activity is associated with increased psychological well-being [16] [17] [18].
Therapies
Mindfulness offers a framework of self-awareness, -regulation, and -transcendence that can be implemented in psychological intervention methods [19]. Mindfulness is now incorporated as a major component of empirically supported psychological intervention methods, including mindfulness-based stress reduction (MSBR) [20], mindfulness-based cognitive therapy (MBCT) [21], acceptance and commitment therapy, and dialectical behavior therapy [22].
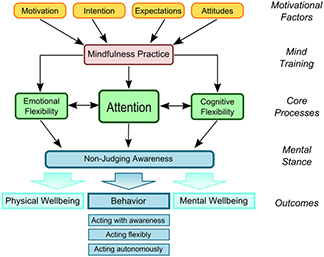
Neurophysiological mechanisms
The cognitive and behavioral effects of mindfulness can be examined through the theoretical framework of emotion regulation. Studies on the neuroscience of mindfulness demonstrate that individuals who engage in mindfulness demonstrate functional and structural differences in the emotional regulation network– specifically, by strengthening the ability to regulate ruminative thinking [23]. Effective emotional regulation facilitates psychological well-being [24].
Brain activity
Early electroencephalogram (EEG) studies and behavioral studies have demonstrated that mindfulness practices engage particular attentional networks. Mindfulness practices produce state changes that increase activation of core nodes in the dorsolateral prefrontal cortex (DLPFC) of the central executive network (CEN) (areas associated with executive function and attention) [25]. Other associated neural networks that show increased activity with mindfulness practices include the anterior subdivision of the cingulate cortex (ACC) [26] and the insular cortex (IC) [27].
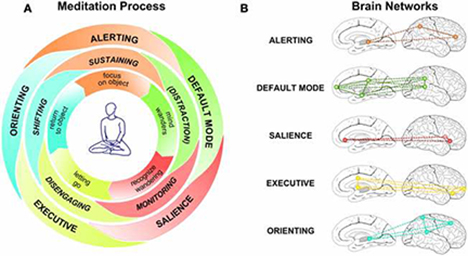
Mindfulness training can result in the upregulation of underlying neural networks in brain activity at a resting state [28], which is correlated with significant improvement in emotion regulation [29]. Causal evidence for neurophysiological mechanisms underlying the cognitive benefits of mindfulness training exist. For example, children who receive mindfulness training intervention exhibit a stronger preserved resting state default mode network (DMN)-DLPFC anticorrelation (decreased activation of the DMN and increased activation in the CEN) compared to controls [30]. Enhanced DMN-DLPFC anticorrelation correlates with better sustained attention performance [31].
Computational theories
Attentional control and emotion regulation are prime targets for computational modeling. However, comprehensive computational frameworks on the effect mindfulness practises have on emotion and cognition are nascent [32].
Bayesian brain hypothesis
One potential computational mechanism that has been proposed to understand the cognitive processes of MBCT is the “Bayesian brain” hypothesis which suggests that the brain creates and continuously update a model of the external world (the physical environment and the physical self) based on input of past experience and homeostatic needs [33]. Neurophysiological studies have begun testing mechanisms proposed by the Bayesian brain hypothesis. For example, EEG studies have demonstrated that mathematical predictions of processes by Bayesian brain theories coincide with brain activity [34] [35] [36]. Predictive coding suggests how cognitive mechanisms are implemented physiologically, which allows for concrete and experimentally testable predictions for the neurophysiological processes affected by MBCT [37]. For example, changes in attentional focus change the precision weighting of sensory channels that are being attended to [38]. Thus, theories in predictive coding coincide with the training phase of MBCT, wherein the practitioner learns to maintain and re-orient attentional focus [39].
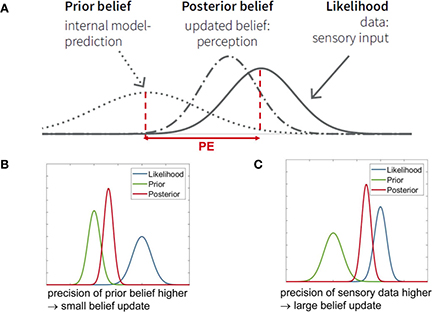
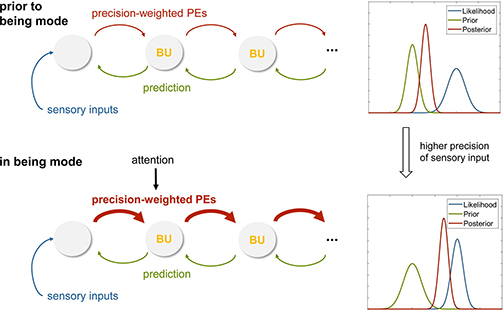
Processes within meditation training, such as MBCT training, can be understood from the perspective of Bayesian models of brain function by deriving experimentally testable predictions between attentional focus and sensory precision. Therefore, computational modeling becomes a clinical tool to obtain subject-specific estimates of precision-weighted prediction errors about physiological states.
Activation propagation model
The activation propagation model aims to explain the information propagation mechanism [40] of the brain (cognitive activity arises from large-scale brain network structure) by modeling neural activity at a macroscopic level, wherein the activity of each brain region at a given time is updated by the amount of input the region receives [41].
The activation propagation model of the brain can be used to simulate how mindfulness practices affect information propagation in the neural network structure of certain brain regions. Neural activity in brain regions can be related to postulates of emotion regulation and behavior.
References
Siegel, R.D., Germer, C.K., Olendzki, A. (2009). Mindfulness: What Is It? Where Did It Come From?. In: Didonna, F. (eds) Clinical Handbook of Mindfulness. Springer, New York, NY. https://doi.org/10.1007/978-0-387-09593-6_2
Hofmann, S. G., Sawyer, A. T., Witt, A. A., & Oh, D. (2010). The effect of mindfulness-based therapy on anxiety and depression: A meta-analytic review. Journal of Consulting and Clinical Psychology, 78(2), 169–183. https://doi.org/10.1037/a0018555
Newberg, A., Alavi, A., Baime, M., Pourdehnad, M., Santanna, J., & d'Aquili, E. (2001). The measurement of regional cerebral blood flow during the complex cognitive task of meditation: a preliminary SPECT study. Psychiatry research, 106(2), 113–122. https://doi.org/10.1016/s0925-4927(01)00074-9
Ritskes, R., Ritskes-Hoitinga, M., Stødkilde-Jørgensen, H., Bærentsen, K., & Hartman, T. (2003). MRI Scanning During Zen Meditation: The Picture of Enlightenment? Constructivism in the Human Sciences, 8(1), 85–90.
Lazar, S. W., Bush, G., Gollub, R. L., Fricchione, G. L., Khalsa, G., & Benson, H. (2000). Functional brain mapping of the relaxation response and meditation. Neuroreport, 11(7), 1581–1585. Malinowski P. (2013). Neural mechanisms of attentional control in mindfulness meditation. Frontiers in neuroscience, 7, 8. https://doi.org/10.3389/fnins.2013.00008
Cásedas, L., Pirruccio, V., Vadillo, M.A. et al. Does Mindfulness Meditation Training Enhance Executive Control? A Systematic Review and Meta-Analysis of Randomized Controlled Trials in Adults. Mindfulness 11, 411–424 (2020). https://doi.org/10.1007/s12671-019-01279-4 Treadway, M. T., & Lazar, S. W. (2009). The neurobiology of mindfulness. In F. Didonna (Ed.), Clinical handbook of mindfulness (pp. 45–57). Springer Science + Business Media. https://doi.org/10.1007/978-0-387-09593-6_4
Miyake, A., Friedman, N. P., Emerson, M. J., Witzki, A. H., Howerter, A., & Wager, T. D. (2000). The unity and diversity of executive functions and their contributions to complex "Frontal Lobe" tasks: a latent variable analysis. Cognitive psychology, 41(1), 49–100. https://doi.org/10.1006/cogp.1999.0734
Yang, Z., Oathes, D. J., Linn, K. A., Bruce, S. E., Satterthwaite, T. D., Cook, P. A., Satchell, E. K., Shou, H., & Sheline, Y. I. (2018). Cognitive Behavioral Therapy Is Associated With Enhanced Cognitive Control Network Activity in Major Depression and Posttraumatic Stress Disorder. Biological psychiatry. Cognitive neuroscience and neuroimaging, 3(4), 311–319. https://doi.org/10.1016/j.bpsc.2017.12.006
Quaglia, J. T., Zeidan, F., Grossenbacher, P. G., Freeman, S. P., Braun, S. E., Martelli, A., Goodman, R. J., & Brown, K. W. (2019). Brief mindfulness training enhances cognitive control in socioemotional contexts: Behavioral and neural evidence. PloS one, 14(7), e0219862. https://doi.org/10.1371/journal.pone.0219862
Kayser, J., Wong, L.Y.X., Sacchi, E. et al. Behavioral measures of attention and cognitive control during a new auditory working memory paradigm. Behav Res 52, 1161–1174 (2020). https://doi.org/10.3758/s13428-019-01308-z Vago, D. R., & Silbersweig, D. A. (2012). Self-awareness, self-regulation, and self-transcendence (S-ART): a framework for understanding the neurobiological mechanisms of mindfulness. Frontiers in human neuroscience, 6, 296. https://doi.org/10.3389/fnhum.2012.00296
Kabat-Zinn J. (1982). An outpatient program in behavioral medicine for chronic pain patients based on the practice of mindfulness meditation: theoretical considerations and preliminary results. General hospital psychiatry, 4(1), 33–47. https://doi.org/10.1016/0163-8343(82)90026-3
Elices, M., Pérez-Sola, V., Pérez-Aranda, A., Colom, F., Polo, M., Martín-López, L. M., & Gárriz, M. (2022). The Effectiveness of Mindfulness-Based Cognitive Therapy in Primary Care and the Role of Depression Severity and Treatment Attendance. Mindfulness, 13(2), 362–372. https://doi.org/10.1007/s12671-021-01794-3
Kabat-Zinn, J. (1982). An outpatient program in behavioral medicine for chronic pain patients based on the practice of mindfulness meditation: theoretical considerations and preliminary results. General Hospital Psychiatry, 4, 33–47. doi:10.1016/0163-8343(82)90026-3.
A. J. Moye and M. K. van Vugt, "A Computational Model of Focused Attention Meditation and Its Transfer to a Sustained Attention Task," in IEEE Transactions on Affective Computing, vol. 12, no. 2, pp. 329-339, 1 April-June 2021, doi: 10.1109/TAFFC.2019.2908172.
Paus T. (2001). Primate anterior cingulate cortex: where motor control, drive and cognition interface. Nature reviews. Neuroscience, 2(6), 417–424. https://doi.org/10.1038/35077500
Brefczynski-Lewis, J. A., Lutz, A., Schaefer, H. S., Levinson, D. B., & Davidson, R. J. (2007). Neural correlates of attentional expertise in long-term meditation practitioners. Proceedings of the National Academy of Sciences of the United States of America, 104(27), 11483–11488. https://doi.org/10.1073/pnas.0606552104
Zhang, Z., Luh, WM., Duan, W. et al. Longitudinal effects of meditation on brain resting-state functional connectivity. Sci Rep 11, 11361 (2021). https://doi.org/10.1038/s41598-021-90729-y Ng, H. H., Wu, C. W., Huang, F. Y., Cheng, Y. T., Guu, S. F., Huang, C. M., Hsu, C. F., Chao, Y. P., Jung, T. P., & Chuang, C. H. (2021). Mindfulness Training Associated With Resting-State Electroencephalograms Dynamics in Novice Practitioners via Mindful Breathing and Body-Scan. Frontiers in psychology, 12, 748584. https://doi.org/10.3389/fpsyg.2021.748584
Bauer, C. C. C., Rozenkrantz, L., Caballero, C., Nieto-Castanon, A., Scherer, E., West, M. R., Mrazek, M., Phillips, D. T., Gabrieli, J. D. E., & Whitfield-Gabrieli, S. (2020). Mindfulness training preserves sustained attention and resting state anticorrelation between default-mode network and dorsolateral prefrontal cortex: A randomized controlled trial. Human brain mapping, 41(18), 5356–5369. https://doi.org/10.1002/hbm.25197
Denkova, E., Nomi, J. S., Uddin, L. Q., & Jha, A. P. (2019). Dynamic brain network configurations during rest and an attention task with frequent occurrence of mind wandering. Human brain mapping, 40(15), 4564–4576. https://doi.org/10.1002/hbm.24721
Manjaly, Z. M., & Iglesias, S. (2020). A Computational Theory of Mindfulness Based Cognitive Therapy from the "Bayesian Brain" Perspective. Frontiers in psychiatry, 11, 404. https://doi.org/10.3389/fpsyt.2020.00404
Gordon, N., Koenig-Robert, R., Tsuchiya, N., van Boxtel, J. J., & Hohwy, J. (2017). Neural markers of predictive coding under perceptual uncertainty revealed with Hierarchical Frequency Tagging. eLife, 6, e22749. https://doi.org/10.7554/eLife.22749
Iglesias, S., Mathys, C., Brodersen, K. H., Kasper, L., Piccirelli, M., den Ouden, H. E., & Stephan, K. E. (2013). Hierarchical prediction errors in midbrain and basal forebrain during sensory learning. Neuron, 80(2), 519–530. https://doi.org/10.1016/j.neuron.2013.09.009
Feldman, H., & Friston, K. J. (2010). Attention, uncertainty, and free-energy. Frontiers in human neuroscience, 4, 215. https://doi.org/10.3389/fnhum.2010.00215
Nakamura, H., Tawatsuji, Y., Fang, S., & Matsui, T. (2021). Explanation of emotion regulation mechanism of mindfulness using a brain function model. Neural networks : the official journal of the International Neural Network Society, 138, 198–214. https://doi.org/10.1016/j.neunet.2021.01.029